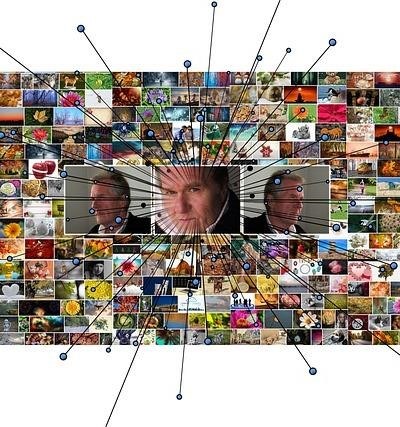
Credit card fraud, particularly CVV2 theft, poses a significant threat to both consumers and businesses. The sheer volume of transactions makes manual fraud detection impractical. Fortunately, advancements in machine learning (ML) offer powerful solutions for enhanced security and fraud prevention.
Understanding the Problem: CVV2 Theft and its Impact
CVV2 (Card Verification Value 2) is a three- or four-digit security code on credit cards designed to protect against unauthorized online purchases. CVV2 theft, often resulting from data breaches or phishing scams, enables fraudsters to make fraudulent transactions. The consequences can be devastating, leading to financial losses, identity theft, and reputational damage.
The Role of Machine Learning in Fraud Detection
Machine learning algorithms, particularly those leveraging anomaly detection and pattern recognition, are revolutionizing fraud detection. These sophisticated systems analyze vast datasets of transaction data, identifying subtle patterns and anomalies indicative of fraudulent activity. This proactive approach is far more effective than relying solely on reactive measures.
Key Machine Learning Techniques:
- Anomaly Detection: ML algorithms identify unusual transaction behaviors that deviate from established norms. This is crucial for detecting CVV2 theft, where fraudulent transactions might exhibit atypical spending patterns or geographical locations.
- Pattern Recognition: By analyzing historical data, ML models can identify recurring patterns associated with successful fraud attempts. This enables predictive modeling, allowing for preemptive risk assessment and the blocking of potentially fraudulent transactions.
- Deep Learning and Neural Networks: These advanced techniques can uncover complex, hidden relationships within the data, leading to more accurate fraud detection. They are particularly effective in analyzing unstructured data, such as transaction descriptions or customer comments.
Implementing Machine Learning for CVV2 Fraud Prevention
Effective implementation requires a multi-faceted approach:
- Data Collection and Preparation: Gathering comprehensive transaction data is paramount. This involves integrating data from various sources, ensuring data quality, and handling missing values.
- Model Development and Training: Selecting appropriate ML algorithms and training them on historical data is crucial for accurate fraud prediction. Regular model retraining is essential to adapt to evolving fraud tactics.
- Transaction Monitoring and Risk Assessment: Real-time transaction monitoring systems, powered by ML models, flag suspicious activity for review; Risk assessment helps prioritize investigations and allocate resources efficiently.
- Fraud Prevention Systems Integration: ML models should be integrated into existing fraud prevention systems to automate alerts, block transactions, and enhance overall security;
Beyond Technology: A Holistic Approach
While technology plays a crucial role, a holistic approach is essential. Strong cybersecurity practices, employee training, and robust data breach response plans are vital components of a comprehensive fraud prevention strategy. Remember, ongoing monitoring, continuous improvement, and adaptation are crucial for staying ahead of evolving fraud techniques.
By leveraging the power of machine learning, organizations can significantly improve their ability to detect and prevent CVV2 theft, safeguarding their customers and their businesses from the devastating consequences of financial crime.
I appreciate the article
An excellent resource for understanding the challenges posed by CVV2 theft and how machine learning is effectively addressing these challenges. The breakdown of key ML techniques, such as anomaly detection and pattern recognition, is particularly helpful. The article successfully highlights the importance of proactive fraud prevention strategies.
A well-written and informative piece that effectively communicates the complexities of credit card fraud and the sophisticated solutions offered by machine learning. The clear and concise language makes it accessible to a broad audience. This article is a valuable asset for anyone seeking to understand the intersection of technology and financial security.
This article provides a clear and concise overview of the critical role machine learning plays in combating credit card fraud, specifically focusing on CVV2 theft. The explanation of the problem and the solutions offered are well-structured and easy to understand, even for those without a deep technical background. Highly recommended for anyone interested in learning about the application of ML in cybersecurity.